7 Vital Tips for Introducing Data Analytics to Your Business
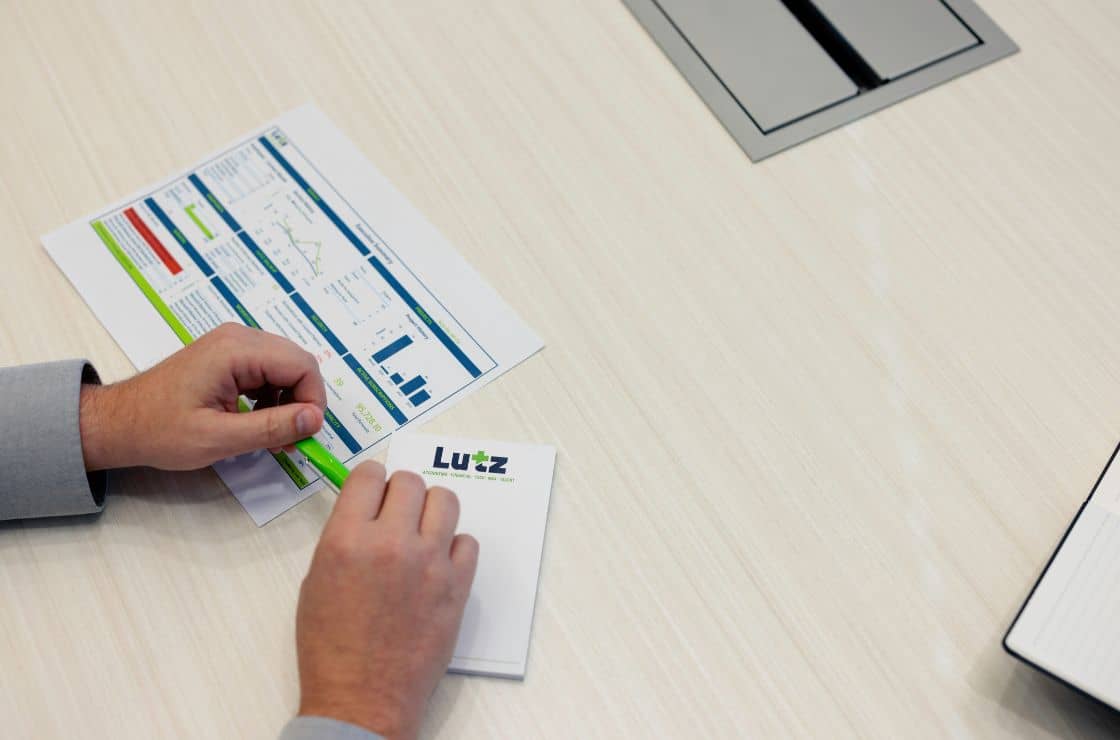
Integrating data analytics into your business can help your revenue grow, the organization mitigate risk or gain insight into your business operations. There's a wealth of information available at your fingertips if you know how to decipher it.
It’s easy to become overloaded figuring out where to start or by the amount of data you have, but it’s important to have a plan and understand your goals before launching the process. Follow these seven tips to make a solid plan for introducing data analytics to your business.
1. What your goals are and why you are introducing analytics.
Understand you and your organization’s meaning of "Analytics."
Data analytics is a term that gets tossed around rather loosely, so be sure it's clearly defined for all those involved. Set realistic expectations and well-defined deliverables, so everyone is on the same page.
The basic definition of data analytics is "the process of analyzing raw data to find trends, patterns, and anomalies to answer questions." Techniques and approaches for producing data analytics can vary widely. It’s important to determine the intended goals before developing a set of results.
Reporting vs. Analytics?
Many organizations wade into “analytics” through some sort of reporting functionality, sometimes referred to as business intelligence. It is important to understand that reports differ from analytics. Reports are simply a static presentation of collected data. Conversely, analytics is a dynamic interpretation of trends and details within that data that indicate reasons for the results in the report and help answer the question of “why?”. Basically, a report is a postmortem display of collected data, while analytics is more of a holistic, real-time explanation of why the results occur, based on granular data points in action.
2. Figure out what business questions you want to answer.
Common missteps in the adoption of analytics involve not asking (or understanding) the true question the business wants to answer or the unrealistic expectation that the data will simply “tell you something” without prompting or direction. The reality is that analytic techniques are applied to help answer specific questions to help explain, identify, validate, or refute a business issue or opportunity.
For example, your organization may say their goal is to use analytics to make more money. However, is that ultimately the question you need to answer? An organization may use analytics to determine, what products are most profitable, how to sell more of those products, what customers buy those products, and how to find or create more of those customers.
The questions your business looks to apply analytics to should focus on those that help them understand the company’s business, customers, products, issues and future opportunities. They usually begin with, "Why did that happen?", and are followed with, "how do we never do that again?" or "how can we do that more often?" The answers to most of these questions may require a multi-step explanation. Make sure you understand the ultimate question management is asking, so you don't chase down a partial answer or fail to identify the “why.”
3. Decide what tools may be needed.
What existing tools might your organization already utilize that can be leveraged?
You might not have to reinvent the wheel. Be sure you understand what's already available to you.
Do you want a system that packages up the analytics already, or will you do some work yourself?
Your journey into data analytics may not be as big of a leap as you think. Check to see if any of your existing systems, like CRM or ERP software, already have automated analytic capabilities embedded. You may want to supplement with a data visualization software like Power BI or Tableau.
4. What skills sets exist within the organization that may be capable of executing or learning data analytic techniques.
Identify folks who have the skills or desire to learn.
There may already be members of your team that have an interest and desire to apply analytics to their day-to-day work. Seek out individuals within the organization that may already be using analytic techniques in their Excel modeling, using data visualization tools or “dabbling” in analytics on the side. Another source can be resources that are involved with your backend databases and have skills around collecting and managing large volumes of data. Make sure you have staff that embraces the possibilities and understands how transformational data analytics can be.
Invest in some training, but…
As you get started, leverage free resources online until you know what tools and skillsets will be most valuable. There are many ways to utilize the data you have available to you, so it's wise to start by dipping your toes in the water before you ramp up capabilities.
5. Identify internal business champions that have a need or desire to use analytics.
These are the people that get it! These partners have a vision and yearning to go beyond the basic nuts and bolts of reporting. Champions of the cause can inspire your whole enterprise to embrace data analytics and help stimulate productive growth.
6. Understand your data landscape.
What data does the organization have?
Make sure you have a good understanding of what data each department collects. To start, shape your analytics program around what's available and plan for collecting and managing more data as the program grows.
Where is the data?
Mature businesses often have legacy systems that have difficulties communicating with each other. Know where to find the data and understand any current limitations to connecting with it. Also, keep in mind that data sources may exist in spreadsheets or other bespoke applications.
How detailed (or not) is the data?
The richer the information, the better the results. When collecting data, try to start with information that is as detailed as possible. Typically, the more, the better. This will allow the most flexibility; however, if aggregated data is all that is available, just make sure the consumer of the analytics understands any limitations that may exist.
How complete (or clean) is the data (Garbage in, garbage out)?
The quality of existing data is crucial to avoid misleading and ineffective analytics. Too often, data input is duplicated or incomplete. Either will skew the workings of the analytics. Data validation and profiling are important to detect potential errors ahead of time. If data is missing or incomplete, come up with a plan to address the handling of any missing information.
As you define the questions you are trying to answer, determining the data landscape is a critical next step.
7. Focus on quick wins to get buy-in from the organization.
You have to crawl before you can run. Early success will fuel more acceptance and appreciation for greater possibilities. Prove your point on a small scale to encourage bigger projects in the future. Grow with the data analytics process. Start with small, manageable projects with clearly defined goals and expectations. Use that experience to plan for future development. Before you know it, management will be suggesting more projects beyond your original scope!
Effective business decisions require sound information and timely data. Properly assembled analytics can bring disparate information together to form logical patterns and associated data points in a more reportable manner that decision-makers can better comprehend. In an age when you're required to "do more with less," effective data analytics can expand the capabilities of a smaller staff.
The possibilities for integrating analytics into your business are endless. These seven steps are a great start to effectively implementing a data analytics program. If you need assistance with your business’s data analytics, contact us today or click here to learn more about our offerings.
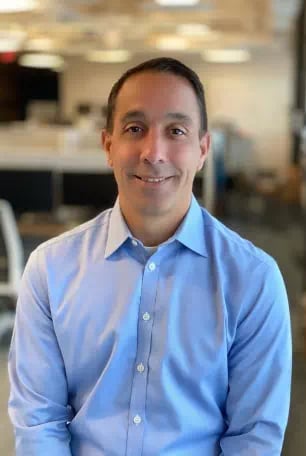
- Maximizer, Deliberative, Consistency, Adaptability, Relator
Tony DeSantis
Tony DeSantis, Data Analytics Shareholder, began his career in 1999. With over two decades of experience in data analytics and forensics, he has honed his skills in interpreting complex data sets and designing impactful visual reports.
Leading Lutz's Data Analytics offering, Tony specializes in data management and the application of artificial intelligence to simplify business processes and create solutions that directly impact their bottom line. He values the firm's unique blend of technical skills and business expertise, which enables them to provide well-rounded solutions to their clients.
At Lutz, Tony is passionate about mentoring and developing emerging talent within the firm. Additionally, he has been actively involved in various policy committees, contributing his expertise to shaping the firm's strategic direction. As the leader of the data analytics team, he consistently strives to make the complex simple for clients, helping them uncover the stories hidden in their information.
Tony lives in Omaha, NE, with his wife and four children. Outside the office, you can find him attending and coaching his kids' sporting events and other activities.
Recent News & Insights
4 Key Components to Individual Income Taxes in Nebraska
Nebraska Business Tax Breakdown
Understanding Working Capital Targets in M&A Transactions
HR Compliance Checklist
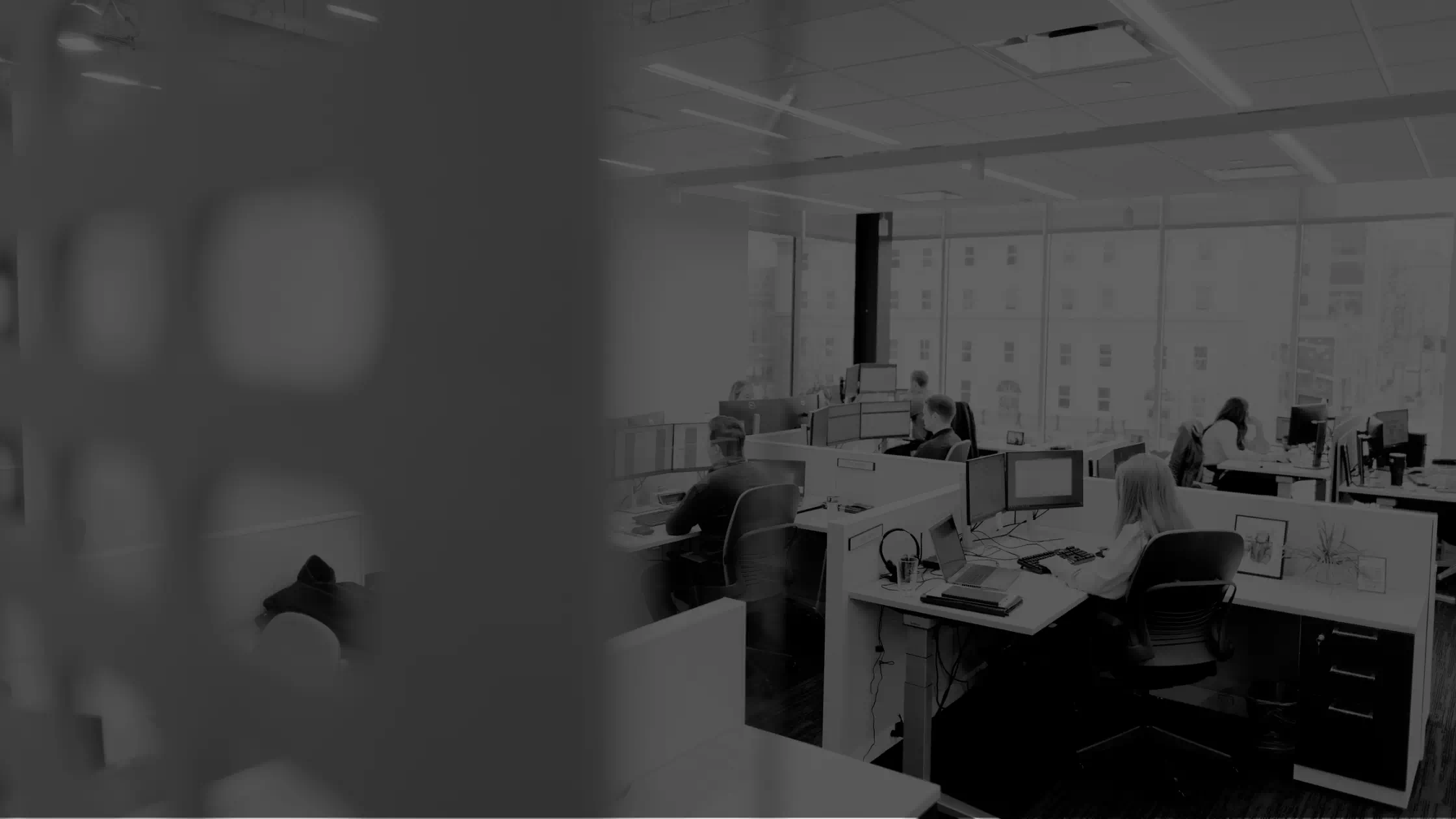
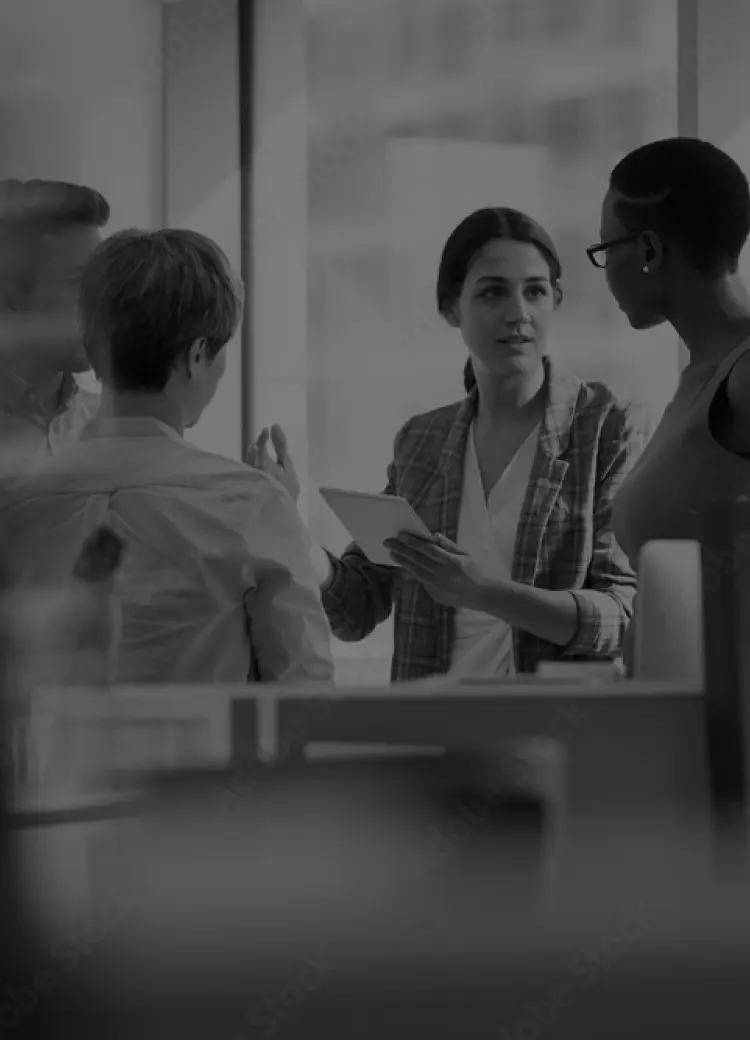